参考:
https://v.qq.com/x/page/e0532hfg6rp.html
https://www.sohu.com/a/161598493_633698
https://www.jianshu.com/p/7ac0e2bba37c
==================================================
I was recently intrigued by Seth Bling’s MarI/O – a neural network slash genetic algorithm that teaches itself to play Super Mario World.
Seth’s implementation (in Lua) is based on the concept of NeuroEvolution of Augmenting Topologies (or NEAT). NEAT is a type of genetic algorithm which generates efficient artificial neural networks (ANNs) from a very simple starting network. It does so rather quickly too (compared to other evolutionary algorithms).

For another example of why this field is incredibly exciting, watch this amazing video of Google’s DeepMind learning and mastering space invaders. How good is that clutch shot at the end?!
Seth’s MarI/O can play both Super Mario World (SNES), and Super Mario Bros (NES). If you want to try it out yourself, read on.
Setup (Windows 8.1)
To evolve your own ANN with MarI/O that can play Super Mario World, here’s how to do it;
Installation
- Install BizHawk Prereqs
- Download and unzip BizHawk
- Get a copy of Seth’s MarI/O (call it neatevolve.lua )
- Put neatevolve.lua in the root folder of your BizHawk folder. (In the same dir as the EmuHawk executable.)
Emulator Setup
- Set BizHawk video Mode to OpenGL (not GDI+)Config > Display > Display Method > Open GL
- Restart BizHawk for settings to take effect. Double check it actually works.
- Optional: Set emulation speed to 200% – this makes the evolution go a lot faster!
Initial State Setup
We need an initial/fresh game state that gets loaded for each genome. In other words, we need to save the ROM state at the start of the desired level we want MarI/O to learn.
- Load the Super Mario World (USA).sfc ROM.
- Start a new game
- Go to the level you want MarI/O to learn. I chose Yoshi’s Island #1.
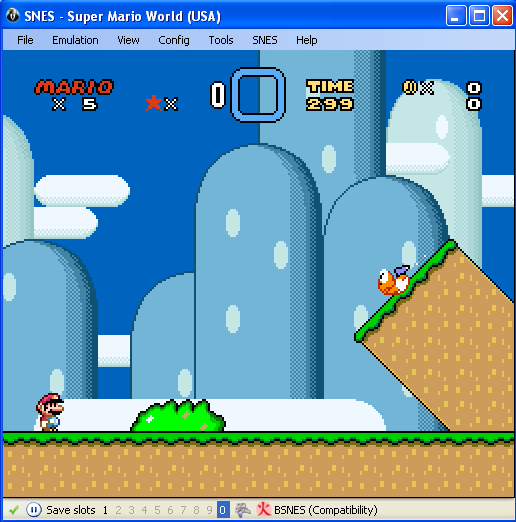
- Use the File -> Save Named State -> Save As “DP1.state” in the BizHawk root folder (i.e. in the same dir as neatevolve.lua).
Now we have an initial state that MarI/O will load before each genome is evaluated.
Running MarI/O
- Load neatevolve.lua. You can do this via Tools->Lua Console. I prefer to drag and drop neatevolve.lua into the running emulator.
- MarI/O will load, creating a base set of about 300 very simple genomes. This is as per the NEAT methodology, which starts with a very simple ANNs (i.e. very few hidden nodes), and evolves from there.
- You can see the ANN that MarI/O is currently evaluating by checking ‘Show Map’ setting in the MarI/O ‘Fitness’ window.
Congratulations! If all goes well you’ll see Mario sitting there or jumping up and down, like an idiot, while it learns how to play the game. Don’t worry, it gets ‘smarter’.
Restarting MarI/O
MarI/O saves the genomes of a given generation in a .pool file. The current generation being evaluated is saved in temp.pool. After each generation, a new .pool file will be saved, prefixed with the generation number.
If your computer melts, and you need to restart MarI/O;
- Delete temp.pool
- Copy the desired generation .pool file to DP1.state.pool
- In the MarI/O ‘Fitness’ window, load the DP1.state.pool
- MarI/O should resume from the latest complete generation.
Troubleshooting
Here are solutions to common errors myself an other people have ran into with MarI/O.
‘Buttonnames’ error
LuaInterface.LuaScriptException: [string "main"]:33: attempt to get length of global 'ButtonNames' (a nil value)
The NEATevolve.lua script has a hardcoded (and relative) file reference to DP1.state. You need to make sure these files are in the same directory.
- Create a Save State in BizHawk at the start of the level you want the algorithm to learn.
- you’ll need to rename that file to DP1.state, and drop it in the same directory as the neatevolve.lua script. Putting both these files in the same directory as EmuHawk.exe is recommended
‘neurons’ error
LuaInterface.LuaScriptException: [string "main"]:337: attempt to index field 'neurons' (a nil value)
A similar error – try the solution above, and failing that;
- As above create a quicksave at the start of a level Renamed the QuickSave1.state found in /SNES/State/ to DP1.state and move it to the folder with the EmuHawk executable.
- Put the neatevolve.lua file in the same folder as EmuHawk.exe.
- Noticed while I was testing that it generated a temp.pool file that seemed to have all the variables in it. Renamed that file to DP1.state.pool
‘Parameter name: source’ error
"System.ArgumentNullException: Value cannot be null. Parameter name: source"
Are you running MarI/O in a VM? Check out my notes on running MarI/O on OSX
Resources
Check out these discussions for more info on MarI/O
========================================
游戏的ROMS文件下载地址:
https://wowroms.com/en/roms/super-nintendo/super-mario-world-usa/29592.html

neatevolve.lua 文件内容:
-- MarI/O by SethBling
-- Feel free to use this code, but please do not redistribute it.
-- Intended for use with the BizHawk emulator and Super Mario World or Super Mario Bros. ROM.
-- For SMW, make sure you have a save state named "DP1.state" at the beginning of a level,
-- and put a copy in both the Lua folder and the root directory of BizHawk.
if gameinfo.getromname() == "Super Mario World (USA)" then
Filename = "DP1.state"
ButtonNames = {
"A",
"B",
"X",
"Y",
"Up",
"Down",
"Left",
"Right",
}
elseif gameinfo.getromname() == "Super Mario Bros." then
Filename = "SMB1-1.state"
ButtonNames = {
"A",
"B",
"Up",
"Down",
"Left",
"Right",
}
end
BoxRadius = 6
InputSize = (BoxRadius*2+1)*(BoxRadius*2+1)
Inputs = InputSize+1
Outputs = #ButtonNames
Population = 300
DeltaDisjoint = 2.0
DeltaWeights = 0.4
DeltaThreshold = 1.0
StaleSpecies = 15
MutateConnectionsChance = 0.25
PerturbChance = 0.90
CrossoverChance = 0.75
LinkMutationChance = 2.0
NodeMutationChance = 0.50
BiasMutationChance = 0.40
StepSize = 0.1
DisableMutationChance = 0.4
EnableMutationChance = 0.2
TimeoutConstant = 20
MaxNodes = 1000000
function getPositions()
if gameinfo.getromname() == "Super Mario World (USA)" then
marioX = memory.read_s16_le(0x94)
marioY = memory.read_s16_le(0x96)
local layer1x = memory.read_s16_le(0x1A);
local layer1y = memory.read_s16_le(0x1C);
screenX = marioX-layer1x
screenY = marioY-layer1y
elseif gameinfo.getromname() == "Super Mario Bros." then
marioX = memory.readbyte(0x6D) * 0x100 + memory.readbyte(0x86)
marioY = memory.readbyte(0x03B8)+16
screenX = memory.readbyte(0x03AD)
screenY = memory.readbyte(0x03B8)
end
end
function getTile(dx, dy)
if gameinfo.getromname() == "Super Mario World (USA)" then
x = math.floor((marioX+dx+8)/16)
y = math.floor((marioY+dy)/16)
return memory.readbyte(0x1C800 + math.floor(x/0x10)*0x1B0 + y*0x10 + x%0x10)
elseif gameinfo.getromname() == "Super Mario Bros." then
local x = marioX + dx + 8
local y = marioY + dy - 16
local page = math.floor(x/256)%2
local subx = math.floor((x%256)/16)
local suby = math.floor((y - 32)/16)
local addr = 0x500 + page*13*16+suby*16+subx
if suby >= 13 or suby < 0 then
return 0
end
if memory.readbyte(addr) ~= 0 then
return 1
else
return 0
end
end
end
function getSprites()
if gameinfo.getromname() == "Super Mario World (USA)" then
local sprites = {}
for slot=0,11 do
local status = memory.readbyte(0x14C8+slot)
if status ~= 0 then
spritex = memory.readbyte(0xE4+slot) + memory.readbyte(0x14E0+slot)*256
spritey = memory.readbyte(0xD8+slot) + memory.readbyte(0x14D4+slot)*256
sprites[#sprites+1] = {["x"]=spritex, ["y"]=spritey}
end
end
return sprites
elseif gameinfo.getromname() == "Super Mario Bros." then
local sprites = {}
for slot=0,4 do
local enemy = memory.readbyte(0xF+slot)
if enemy ~= 0 then
local ex = memory.readbyte(0x6E + slot)*0x100 + memory.readbyte(0x87+slot)
local ey = memory.readbyte(0xCF + slot)+24
sprites[#sprites+1] = {["x"]=ex,["y"]=ey}
end
end
return sprites
end
end
function getExtendedSprites()
if gameinfo.getromname() == "Super Mario World (USA)" then
local extended = {}
for slot=0,11 do
local number = memory.readbyte(0x170B+slot)
if number ~= 0 then
spritex = memory.readbyte(0x171F+slot) + memory.readbyte(0x1733+slot)*256
spritey = memory.readbyte(0x1715+slot) + memory.readbyte(0x1729+slot)*256
extended[#extended+1] = {["x"]=spritex, ["y"]=spritey}
end
end
return extended
elseif gameinfo.getromname() == "Super Mario Bros." then
return {}
end
end
function getInputs()
getPositions()
sprites = getSprites()
extended = getExtendedSprites()
local inputs = {}
for dy=-BoxRadius*16,BoxRadius*16,16 do
for dx=-BoxRadius*16,BoxRadius*16,16 do
inputs[#inputs+1] = 0
tile = getTile(dx, dy)
if tile == 1 and marioY+dy < 0x1B0 then
inputs[#inputs] = 1
end
for i = 1,#sprites do
distx = math.abs(sprites[i]["x"] - (marioX+dx))
disty = math.abs(sprites[i]["y"] - (marioY+dy))
if distx <= 8 and disty <= 8 then
inputs[#inputs] = -1
end
end
for i = 1,#extended do
distx = math.abs(extended[i]["x"] - (marioX+dx))
disty = math.abs(extended[i]["y"] - (marioY+dy))
if distx < 8 and disty < 8 then
inputs[#inputs] = -1
end
end
end
end
--mariovx = memory.read_s8(0x7B)
--mariovy = memory.read_s8(0x7D)
return inputs
end
function sigmoid(x)
return 2/(1+math.exp(-4.9*x))-1
end
function newInnovation()
pool.innovation = pool.innovation + 1
return pool.innovation
end
function newPool()
local pool = {}
pool.species = {}
pool.generation = 0
pool.innovation = Outputs
pool.currentSpecies = 1
pool.currentGenome = 1
pool.currentFrame = 0
pool.maxFitness = 0
return pool
end
function newSpecies()
local species = {}
species.topFitness = 0
species.staleness = 0
species.genomes = {}
species.averageFitness = 0
return species
end
function newGenome()
local genome = {}
genome.genes = {}
genome.fitness = 0
genome.adjustedFitness = 0
genome.network = {}
genome.maxneuron = 0
genome.globalRank = 0
genome.mutationRates = {}
genome.mutationRates["connections"] = MutateConnectionsChance
genome.mutationRates["link"] = LinkMutationChance
genome.mutationRates["bias"] = BiasMutationChance
genome.mutationRates["node"] = NodeMutationChance
genome.mutationRates["enable"] = EnableMutationChance
genome.mutationRates["disable"] = DisableMutationChance
genome.mutationRates["step"] = StepSize
return genome
end
function copyGenome(genome)
local genome2 = newGenome()
for g=1,#genome.genes do
table.insert(genome2.genes, copyGene(genome.genes[g]))
end
genome2.maxneuron = genome.maxneuron
genome2.mutationRates["connections"] = genome.mutationRates["connections"]
genome2.mutationRates["link"] = genome.mutationRates["link"]
genome2.mutationRates["bias"] = genome.mutationRates["bias"]
genome2.mutationRates["node"] = genome.mutationRates["node"]
genome2.mutationRates["enable"] = genome.mutationRates["enable"]
genome2.mutationRates["disable"] = genome.mutationRates["disable"]
return genome2
end
function basicGenome()
local genome = newGenome()
local innovation = 1
genome.maxneuron = Inputs
mutate(genome)
return genome
end
function newGene()
local gene = {}
gene.into = 0
gene.out = 0
gene.weight = 0.0
gene.enabled = true
gene.innovation = 0
return gene
end
function copyGene(gene)
local gene2 = newGene()
gene2.into = gene.into
gene2.out = gene.out
gene2.weight = gene.weight
gene2.enabled = gene.enabled
gene2.innovation = gene.innovation
return gene2
end
function newNeuron()
local neuron = {}
neuron.incoming = {}
neuron.value = 0.0
return neuron
end
function generateNetwork(genome)
local network = {}
network.neurons = {}
for i=1,Inputs do
network.neurons[i] = newNeuron()
end
for o=1,Outputs do
network.neurons[MaxNodes+o] = newNeuron()
end
table.sort(genome.genes, function (a,b)
return (a.out < b.out)
end)
for i=1,#genome.genes do
local gene = genome.genes[i]
if gene.enabled then
if network.neurons[gene.out] == nil then
network.neurons[gene.out] = newNeuron()
end
local neuron = network.neurons[gene.out]
table.insert(neuron.incoming, gene)
if network.neurons[gene.into] == nil then
network.neurons[gene.into] = newNeuron()
end
end
end
genome.network = network
end
function evaluateNetwork(network, inputs)
table.insert(inputs, 1)
if #inputs ~= Inputs then
console.writeline("Incorrect number of neural network inputs.")
return {}
end
for i=1,Inputs do
network.neurons[i].value = inputs[i]
end
for _,neuron in pairs(network.neurons) do
local sum = 0
for j = 1,#neuron.incoming do
local incoming = neuron.incoming[j]
local other = network.neurons[incoming.into]
sum = sum + incoming.weight * other.value
end
if #neuron.incoming > 0 then
neuron.value = sigmoid(sum)
end
end
local outputs = {}
for o=1,Outputs do
local button = "P1 " .. ButtonNames[o]
if network.neurons[MaxNodes+o].value > 0 then
outputs[button] = true
else
outputs[button] = false
end
end
return outputs
end
function crossover(g1, g2)
-- Make sure g1 is the higher fitness genome
if g2.fitness > g1.fitness then
tempg = g1
g1 = g2
g2 = tempg
end
local child = newGenome()
local innovations2 = {}
for i=1,#g2.genes do
local gene = g2.genes[i]
innovations2[gene.innovation] = gene
end
for i=1,#g1.genes do
local gene1 = g1.genes[i]
local gene2 = innovations2[gene1.innovation]
if gene2 ~= nil and math.random(2) == 1 and gene2.enabled then
table.insert(child.genes, copyGene(gene2))
else
table.insert(child.genes, copyGene(gene1))
end
end
child.maxneuron = math.max(g1.maxneuron,g2.maxneuron)
for mutation,rate in pairs(g1.mutationRates) do
child.mutationRates[mutation] = rate
end
return child
end
function randomNeuron(genes, nonInput)
local neurons = {}
if not nonInput then
for i=1,Inputs do
neurons[i] = true
end
end
for o=1,Outputs do
neurons[MaxNodes+o] = true
end
for i=1,#genes do
if (not nonInput) or genes[i].into > Inputs then
neurons[genes[i].into] = true
end
if (not nonInput) or genes[i].out > Inputs then
neurons[genes[i].out] = true
end
end
local count = 0
for _,_ in pairs(neurons) do
count = count + 1
end
local n = math.random(1, count)
for k,v in pairs(neurons) do
n = n-1
if n == 0 then
return k
end
end
return 0
end
function containsLink(genes, link)
for i=1,#genes do
local gene = genes[i]
if gene.into == link.into and gene.out == link.out then
return true
end
end
end
function pointMutate(genome)
local step = genome.mutationRates["step"]
for i=1,#genome.genes do
local gene = genome.genes[i]
if math.random() < PerturbChance then
gene.weight = gene.weight + math.random() * step*2 - step
else
gene.weight = math.random()*4-2
end
end
end
function linkMutate(genome, forceBias)
local neuron1 = randomNeuron(genome.genes, false)
local neuron2 = randomNeuron(genome.genes, true)
local newLink = newGene()
if neuron1 <= Inputs and neuron2 <= Inputs then
--Both input nodes
return
end
if neuron2 <= Inputs then
-- Swap output and input
local temp = neuron1
neuron1 = neuron2
neuron2 = temp
end
newLink.into = neuron1
newLink.out = neuron2
if forceBias then
newLink.into = Inputs
end
if containsLink(genome.genes, newLink) then
return
end
newLink.innovation = newInnovation()
newLink.weight = math.random()*4-2
table.insert(genome.genes, newLink)
end
function nodeMutate(genome)
if #genome.genes == 0 then
return
end
genome.maxneuron = genome.maxneuron + 1
local gene = genome.genes[math.random(1,#genome.genes)]
if not gene.enabled then
return
end
gene.enabled = false
local gene1 = copyGene(gene)
gene1.out = genome.maxneuron
gene1.weight = 1.0
gene1.innovation = newInnovation()
gene1.enabled = true
table.insert(genome.genes, gene1)
local gene2 = copyGene(gene)
gene2.into = genome.maxneuron
gene2.innovation = newInnovation()
gene2.enabled = true
table.insert(genome.genes, gene2)
end
function enableDisableMutate(genome, enable)
local candidates = {}
for _,gene in pairs(genome.genes) do
if gene.enabled == not enable then
table.insert(candidates, gene)
end
end
if #candidates == 0 then
return
end
local gene = candidates[math.random(1,#candidates)]
gene.enabled = not gene.enabled
end
function mutate(genome)
for mutation,rate in pairs(genome.mutationRates) do
if math.random(1,2) == 1 then
genome.mutationRates[mutation] = 0.95*rate
else
genome.mutationRates[mutation] = 1.05263*rate
end
end
if math.random() < genome.mutationRates["connections"] then
pointMutate(genome)
end
local p = genome.mutationRates["link"]
while p > 0 do
if math.random() < p then
linkMutate(genome, false)
end
p = p - 1
end
p = genome.mutationRates["bias"]
while p > 0 do
if math.random() < p then
linkMutate(genome, true)
end
p = p - 1
end
p = genome.mutationRates["node"]
while p > 0 do
if math.random() < p then
nodeMutate(genome)
end
p = p - 1
end
p = genome.mutationRates["enable"]
while p > 0 do
if math.random() < p then
enableDisableMutate(genome, true)
end
p = p - 1
end
p = genome.mutationRates["disable"]
while p > 0 do
if math.random() < p then
enableDisableMutate(genome, false)
end
p = p - 1
end
end
function disjoint(genes1, genes2)
local i1 = {}
for i = 1,#genes1 do
local gene = genes1[i]
i1[gene.innovation] = true
end
local i2 = {}
for i = 1,#genes2 do
local gene = genes2[i]
i2[gene.innovation] = true
end
local disjointGenes = 0
for i = 1,#genes1 do
local gene = genes1[i]
if not i2[gene.innovation] then
disjointGenes = disjointGenes+1
end
end
for i = 1,#genes2 do
local gene = genes2[i]
if not i1[gene.innovation] then
disjointGenes = disjointGenes+1
end
end
local n = math.max(#genes1, #genes2)
return disjointGenes / n
end
function weights(genes1, genes2)
local i2 = {}
for i = 1,#genes2 do
local gene = genes2[i]
i2[gene.innovation] = gene
end
local sum = 0
local coincident = 0
for i = 1,#genes1 do
local gene = genes1[i]
if i2[gene.innovation] ~= nil then
local gene2 = i2[gene.innovation]
sum = sum + math.abs(gene.weight - gene2.weight)
coincident = coincident + 1
end
end
return sum / coincident
end
function sameSpecies(genome1, genome2)
local dd = DeltaDisjoint*disjoint(genome1.genes, genome2.genes)
local dw = DeltaWeights*weights(genome1.genes, genome2.genes)
return dd + dw < DeltaThreshold
end
function rankGlobally()
local global = {}
for s = 1,#pool.species do
local species = pool.species[s]
for g = 1,#species.genomes do
table.insert(global, species.genomes[g])
end
end
table.sort(global, function (a,b)
return (a.fitness < b.fitness)
end)
for g=1,#global do
global[g].globalRank = g
end
end
function calculateAverageFitness(species)
local total = 0
for g=1,#species.genomes do
local genome = species.genomes[g]
total = total + genome.globalRank
end
species.averageFitness = total / #species.genomes
end
function totalAverageFitness()
local total = 0
for s = 1,#pool.species do
local species = pool.species[s]
total = total + species.averageFitness
end
return total
end
function cullSpecies(cutToOne)
for s = 1,#pool.species do
local species = pool.species[s]
table.sort(species.genomes, function (a,b)
return (a.fitness > b.fitness)
end)
local remaining = math.ceil(#species.genomes/2)
if cutToOne then
remaining = 1
end
while #species.genomes > remaining do
table.remove(species.genomes)
end
end
end
function breedChild(species)
local child = {}
if math.random() < CrossoverChance then
g1 = species.genomes[math.random(1, #species.genomes)]
g2 = species.genomes[math.random(1, #species.genomes)]
child = crossover(g1, g2)
else
g = species.genomes[math.random(1, #species.genomes)]
child = copyGenome(g)
end
mutate(child)
return child
end
function removeStaleSpecies()
local survived = {}
for s = 1,#pool.species do
local species = pool.species[s]
table.sort(species.genomes, function (a,b)
return (a.fitness > b.fitness)
end)
if species.genomes[1].fitness > species.topFitness then
species.topFitness = species.genomes[1].fitness
species.staleness = 0
else
species.staleness = species.staleness + 1
end
if species.staleness < StaleSpecies or species.topFitness >= pool.maxFitness then
table.insert(survived, species)
end
end
pool.species = survived
end
function removeWeakSpecies()
local survived = {}
local sum = totalAverageFitness()
for s = 1,#pool.species do
local species = pool.species[s]
breed = math.floor(species.averageFitness / sum * Population)
if breed >= 1 then
table.insert(survived, species)
end
end
pool.species = survived
end
function addToSpecies(child)
local foundSpecies = false
for s=1,#pool.species do
local species = pool.species[s]
if not foundSpecies and sameSpecies(child, species.genomes[1]) then
table.insert(species.genomes, child)
foundSpecies = true
end
end
if not foundSpecies then
local childSpecies = newSpecies()
table.insert(childSpecies.genomes, child)
table.insert(pool.species, childSpecies)
end
end
function newGeneration()
cullSpecies(false) -- Cull the bottom half of each species
rankGlobally()
removeStaleSpecies()
rankGlobally()
for s = 1,#pool.species do
local species = pool.species[s]
calculateAverageFitness(species)
end
removeWeakSpecies()
local sum = totalAverageFitness()
local children = {}
for s = 1,#pool.species do
local species = pool.species[s]
breed = math.floor(species.averageFitness / sum * Population) - 1
for i=1,breed do
table.insert(children, breedChild(species))
end
end
cullSpecies(true) -- Cull all but the top member of each species
while #children + #pool.species < Population do
local species = pool.species[math.random(1, #pool.species)]
table.insert(children, breedChild(species))
end
for c=1,#children do
local child = children[c]
addToSpecies(child)
end
pool.generation = pool.generation + 1
writeFile("backup." .. pool.generation .. "." .. forms.gettext(saveLoadFile))
end
function initializePool()
pool = newPool()
for i=1,Population do
basic = basicGenome()
addToSpecies(basic)
end
initializeRun()
end
function clearJoypad()
controller = {}
for b = 1,#ButtonNames do
controller["P1 " .. ButtonNames[b]] = false
end
joypad.set(controller)
end
function initializeRun()
savestate.load(Filename);
rightmost = 0
pool.currentFrame = 0
timeout = TimeoutConstant
clearJoypad()
local species = pool.species[pool.currentSpecies]
local genome = species.genomes[pool.currentGenome]
generateNetwork(genome)
evaluateCurrent()
end
function evaluateCurrent()
local species = pool.species[pool.currentSpecies]
local genome = species.genomes[pool.currentGenome]
inputs = getInputs()
controller = evaluateNetwork(genome.network, inputs)
if controller["P1 Left"] and controller["P1 Right"] then
controller["P1 Left"] = false
controller["P1 Right"] = false
end
if controller["P1 Up"] and controller["P1 Down"] then
controller["P1 Up"] = false
controller["P1 Down"] = false
end
joypad.set(controller)
end
if pool == nil then
initializePool()
end
function nextGenome()
pool.currentGenome = pool.currentGenome + 1
if pool.currentGenome > #pool.species[pool.currentSpecies].genomes then
pool.currentGenome = 1
pool.currentSpecies = pool.currentSpecies+1
if pool.currentSpecies > #pool.species then
newGeneration()
pool.currentSpecies = 1
end
end
end
function fitnessAlreadyMeasured()
local species = pool.species[pool.currentSpecies]
local genome = species.genomes[pool.currentGenome]
return genome.fitness ~= 0
end
function displayGenome(genome)
local network = genome.network
local cells = {}
local i = 1
local cell = {}
for dy=-BoxRadius,BoxRadius do
for dx=-BoxRadius,BoxRadius do
cell = {}
cell.x = 50+5*dx
cell.y = 70+5*dy
cell.value = network.neurons[i].value
cells[i] = cell
i = i + 1
end
end
local biasCell = {}
biasCell.x = 80
biasCell.y = 110
biasCell.value = network.neurons[Inputs].value
cells[Inputs] = biasCell
for o = 1,Outputs do
cell = {}
cell.x = 220
cell.y = 30 + 8 * o
cell.value = network.neurons[MaxNodes + o].value
cells[MaxNodes+o] = cell
local color
if cell.value > 0 then
color = 0xFF0000FF
else
color = 0xFF000000
end
gui.drawText(223, 24+8*o, ButtonNames[o], color, 9)
end
for n,neuron in pairs(network.neurons) do
cell = {}
if n > Inputs and n <= MaxNodes then
cell.x = 140
cell.y = 40
cell.value = neuron.value
cells[n] = cell
end
end
for n=1,4 do
for _,gene in pairs(genome.genes) do
if gene.enabled then
local c1 = cells[gene.into]
local c2 = cells[gene.out]
if gene.into > Inputs and gene.into <= MaxNodes then
c1.x = 0.75*c1.x + 0.25*c2.x
if c1.x >= c2.x then
c1.x = c1.x - 40
end
if c1.x < 90 then
c1.x = 90
end
if c1.x > 220 then
c1.x = 220
end
c1.y = 0.75*c1.y + 0.25*c2.y
end
if gene.out > Inputs and gene.out <= MaxNodes then
c2.x = 0.25*c1.x + 0.75*c2.x
if c1.x >= c2.x then
c2.x = c2.x + 40
end
if c2.x < 90 then
c2.x = 90
end
if c2.x > 220 then
c2.x = 220
end
c2.y = 0.25*c1.y + 0.75*c2.y
end
end
end
end
gui.drawBox(50-BoxRadius*5-3,70-BoxRadius*5-3,50+BoxRadius*5+2,70+BoxRadius*5+2,0xFF000000, 0x80808080)
for n,cell in pairs(cells) do
if n > Inputs or cell.value ~= 0 then
local color = math.floor((cell.value+1)/2*256)
if color > 255 then color = 255 end
if color < 0 then color = 0 end
local opacity = 0xFF000000
if cell.value == 0 then
opacity = 0x50000000
end
color = opacity + color*0x10000 + color*0x100 + color
gui.drawBox(cell.x-2,cell.y-2,cell.x+2,cell.y+2,opacity,color)
end
end
for _,gene in pairs(genome.genes) do
if gene.enabled then
local c1 = cells[gene.into]
local c2 = cells[gene.out]
local opacity = 0xA0000000
if c1.value == 0 then
opacity = 0x20000000
end
local color = 0x80-math.floor(math.abs(sigmoid(gene.weight))*0x80)
if gene.weight > 0 then
color = opacity + 0x8000 + 0x10000*color
else
color = opacity + 0x800000 + 0x100*color
end
gui.drawLine(c1.x+1, c1.y, c2.x-3, c2.y, color)
end
end
gui.drawBox(49,71,51,78,0x00000000,0x80FF0000)
if forms.ischecked(showMutationRates) then
local pos = 100
for mutation,rate in pairs(genome.mutationRates) do
gui.drawText(100, pos, mutation .. ": " .. rate, 0xFF000000, 10)
pos = pos + 8
end
end
end
function writeFile(filename)
local file = io.open(filename, "w")
file:write(pool.generation .. "\n")
file:write(pool.maxFitness .. "\n")
file:write(#pool.species .. "\n")
for n,species in pairs(pool.species) do
file:write(species.topFitness .. "\n")
file:write(species.staleness .. "\n")
file:write(#species.genomes .. "\n")
for m,genome in pairs(species.genomes) do
file:write(genome.fitness .. "\n")
file:write(genome.maxneuron .. "\n")
for mutation,rate in pairs(genome.mutationRates) do
file:write(mutation .. "\n")
file:write(rate .. "\n")
end
file:write("done\n")
file:write(#genome.genes .. "\n")
for l,gene in pairs(genome.genes) do
file:write(gene.into .. " ")
file:write(gene.out .. " ")
file:write(gene.weight .. " ")
file:write(gene.innovation .. " ")
if(gene.enabled) then
file:write("1\n")
else
file:write("0\n")
end
end
end
end
file:close()
end
function savePool()
local filename = forms.gettext(saveLoadFile)
writeFile(filename)
end
function loadFile(filename)
local file = io.open(filename, "r")
pool = newPool()
pool.generation = file:read("*number")
pool.maxFitness = file:read("*number")
forms.settext(maxFitnessLabel, "Max Fitness: " .. math.floor(pool.maxFitness))
local numSpecies = file:read("*number")
for s=1,numSpecies do
local species = newSpecies()
table.insert(pool.species, species)
species.topFitness = file:read("*number")
species.staleness = file:read("*number")
local numGenomes = file:read("*number")
for g=1,numGenomes do
local genome = newGenome()
table.insert(species.genomes, genome)
genome.fitness = file:read("*number")
genome.maxneuron = file:read("*number")
local line = file:read("*line")
while line ~= "done" do
genome.mutationRates[line] = file:read("*number")
line = file:read("*line")
end
local numGenes = file:read("*number")
for n=1,numGenes do
local gene = newGene()
table.insert(genome.genes, gene)
local enabled
gene.into, gene.out, gene.weight, gene.innovation, enabled = file:read("*number", "*number", "*number", "*number", "*number")
if enabled == 0 then
gene.enabled = false
else
gene.enabled = true
end
end
end
end
file:close()
while fitnessAlreadyMeasured() do
nextGenome()
end
initializeRun()
pool.currentFrame = pool.currentFrame + 1
end
function loadPool()
local filename = forms.gettext(saveLoadFile)
loadFile(filename)
end
function playTop()
local maxfitness = 0
local maxs, maxg
for s,species in pairs(pool.species) do
for g,genome in pairs(species.genomes) do
if genome.fitness > maxfitness then
maxfitness = genome.fitness
maxs = s
maxg = g
end
end
end
pool.currentSpecies = maxs
pool.currentGenome = maxg
pool.maxFitness = maxfitness
forms.settext(maxFitnessLabel, "Max Fitness: " .. math.floor(pool.maxFitness))
initializeRun()
pool.currentFrame = pool.currentFrame + 1
return
end
function onExit()
forms.destroy(form)
end
writeFile("temp.pool")
event.onexit(onExit)
form = forms.newform(200, 260, "Fitness")
maxFitnessLabel = forms.label(form, "Max Fitness: " .. math.floor(pool.maxFitness), 5, 8)
showNetwork = forms.checkbox(form, "Show Map", 5, 30)
showMutationRates = forms.checkbox(form, "Show M-Rates", 5, 52)
restartButton = forms.button(form, "Restart", initializePool, 5, 77)
saveButton = forms.button(form, "Save", savePool, 5, 102)
loadButton = forms.button(form, "Load", loadPool, 80, 102)
saveLoadFile = forms.textbox(form, Filename .. ".pool", 170, 25, nil, 5, 148)
saveLoadLabel = forms.label(form, "Save/Load:", 5, 129)
playTopButton = forms.button(form, "Play Top", playTop, 5, 170)
hideBanner = forms.checkbox(form, "Hide Banner", 5, 190)
while true do
local backgroundColor = 0xD0FFFFFF
if not forms.ischecked(hideBanner) then
gui.drawBox(0, 0, 300, 26, backgroundColor, backgroundColor)
end
local species = pool.species[pool.currentSpecies]
local genome = species.genomes[pool.currentGenome]
if forms.ischecked(showNetwork) then
displayGenome(genome)
end
if pool.currentFrame%5 == 0 then
evaluateCurrent()
end
joypad.set(controller)
getPositions()
if marioX > rightmost then
rightmost = marioX
timeout = TimeoutConstant
end
timeout = timeout - 1
local timeoutBonus = pool.currentFrame / 4
if timeout + timeoutBonus <= 0 then
local fitness = rightmost - pool.currentFrame / 2
if gameinfo.getromname() == "Super Mario World (USA)" and rightmost > 4816 then
fitness = fitness + 1000
end
if gameinfo.getromname() == "Super Mario Bros." and rightmost > 3186 then
fitness = fitness + 1000
end
if fitness == 0 then
fitness = -1
end
genome.fitness = fitness
if fitness > pool.maxFitness then
pool.maxFitness = fitness
forms.settext(maxFitnessLabel, "Max Fitness: " .. math.floor(pool.maxFitness))
writeFile("backup." .. pool.generation .. "." .. forms.gettext(saveLoadFile))
end
console.writeline("Gen " .. pool.generation .. " species " .. pool.currentSpecies .. " genome " .. pool.currentGenome .. " fitness: " .. fitness)
pool.currentSpecies = 1
pool.currentGenome = 1
while fitnessAlreadyMeasured() do
nextGenome()
end
initializeRun()
end
local measured = 0
local total = 0
for _,species in pairs(pool.species) do
for _,genome in pairs(species.genomes) do
total = total + 1
if genome.fitness ~= 0 then
measured = measured + 1
end
end
end
if not forms.ischecked(hideBanner) then
gui.drawText(0, 0, "Gen " .. pool.generation .. " species " .. pool.currentSpecies .. " genome " .. pool.currentGenome .. " (" .. math.floor(measured/total*100) .. "%)", 0xFF000000, 11)
gui.drawText(0, 12, "Fitness: " .. math.floor(rightmost - (pool.currentFrame) / 2 - (timeout + timeoutBonus)*2/3), 0xFF000000, 11)
gui.drawText(100, 12, "Max Fitness: " .. math.floor(pool.maxFitness), 0xFF000000, 11)
end
pool.currentFrame = pool.currentFrame + 1
emu.frameadvance();
end
==========================================================
注意:
neatevolve.lua 文件 和 DP1.State 需要放在同一目录下,不然的话执行lua脚本时会找不到游戏的起始状态文件(DP1.State)。
Super Mario World (USA).sfc 游戏文件的位置没有特殊要求,本人操作时为了方便便将其一并放在了模拟器的根目录中。

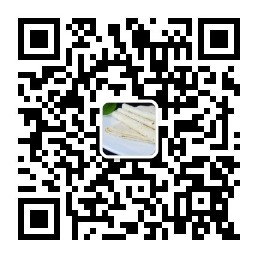