Extreme Parkour with Legged Robots
X Cheng, K Shi, A Agarwal, D Pathak
[CMU]
四足机器人极限跑酷
- 本文训练四足机器人直接从深度图像进行极限跑酷动作,如长跳、高跳和手倒立。
- 主要挑战是在低成本机器人上利用不精确的感知和驱动获得精确控制,通过模拟中的强化学习和双重蒸馏方法将模型迁移到现实世界。
- 提出基于内积的统一奖励设计,可以自动获得不同的运动技巧,如手倒立,机器人可以无缝地在四足和二足步态之间转换。
- 结果显示,机器人可以进行高度是自己身高两倍(0.5米)的高跳,跨度是自己身长两倍(0.8米)的长跳,以及二足手倒立步行。
- 与使用高程图、形状奖励和人为指定运行方向的基准方法相比,本文方法取得更好表现,并可以推广到新环境,而经典模块化方法不可以。
动机:传统的机器人在进行极限跑酷动作时需要独立设计感知、驱动和控制系统,限制了它们在受控环境外的应用。相比之下,人类能通过练习学会跑酷动作,而不需要改变其生物学特性。本文旨在通过在低成本机器人上使用单一神经网络来开发机器人跑酷,克服感知和驱动不精确的挑战。
方法:通过在仿真中使用大规模强化学习训练单一神经网络,直接从摄像头图像中输出高精度的控制行为。在部署时,使用了一种新的双重蒸馏方法来训练神经网络调整自身的前进方向。此外,还提出一种基于内积的简单而有效的统一奖励设计原则,用于获取机器人的基本运动技能。
优势:使用低成本机器人和单一神经网络,通过强化学习训练,实现了在极限跑酷中精确的控制行为。该方法能克服感知和驱动不准确的挑战,使机器人能在不同的物理环境中执行跑酷动作。
一句话总结:
通过在低成本机器人上使用单一神经网络,通过强化学习训练实现在极限跑酷中精确的控制行为,克服感知和驱动不准确的挑战。
Humans can perform parkour by traversing obstacles in a highly dynamic fashion requiring precise eye-muscle coordination and movement. Getting robots to do the same task requires overcoming similar challenges. Classically, this is done by independently engineering perception, actuation, and control systems to very low tolerances. This restricts them to tightly controlled settings such as a predetermined obstacle course in labs. In contrast, humans are able to learn parkour through practice without significantly changing their underlying biology. In this paper, we take a similar approach to developing robot parkour on a small low-cost robot with imprecise actuation and a single front-facing depth camera for perception which is low-frequency, jittery, and prone to artifacts. We show how a single neural net policy operating directly from a camera image, trained in simulation with largescale RL, can overcome imprecise sensing and actuation to output highly precise control behavior end-to-end. We show our robot can perform a high jump on obstacles 2x its height, long jump across gaps 2x its length, do a handstand and run across tilted ramps, and generalize to novel obstacle courses with different physical properties. Parkour videos at https://extreme-parkour.github.io/
https://arxiv.org/abs/2309.14341
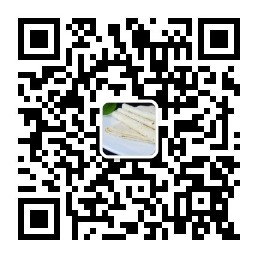