今天是2023年10月8日,星期日,北京,天气晴,我们再来看看大模型在RAG行业问答落地中的一些观点和实践。
实际上,在真实落地中,RAG存在几个比较突出的问题,我们社区对此也进行了不少讨论,总结了下,主要包括三个点:
第一,RAG进行应用权衡文档长度和返回文档数据的问题。这个本质上是召回数据相关性的问题。而召回数据相关性的影响方面很多,既包括文档的切分,也包括文档query输入的清晰度,因此现在也出现了从多query、多召回策略以及排序修正等多个方案。针对这个问题,我们会来讲讲最近的一个方法,RAG-Fusion和RRF。
第二,关于问答答案散落在多个文档以及多个文档内部段落里的问题,这个问题的根在于,在有限的上下文窗口内,不同的答案很分散,不容易都放在窗口之内,因此需要将文本进行聚类(主题分割),尽可能地让有限的窗口下能塞满多个主题的内容。
因此,我们会有很多思路,例如前面说到的泛化query来召回不同的答案点,又如,通过主题分割、检索文本主题聚类等方法进行处理。
第三,引文生成的问题。现有的RAG方案都是先召回一堆文档,然后让大模型根据文档进行作答,但其中更重要的是能够自动的生成引文角标,如下图所示。
我们之前在《引证生成让大模型问答结果更为可信:也看大型语言模型生成带引证的文本方法与评估基准ALCE》一文中,介绍了最近的工作《Enabling Large Language Models to Generate Text with Citations (使大型语言模型生成带引证的文本)》,里面提到的方案可供参考,可以通过in-context learning,也可以通过微调,让大模型自动生成引文。
但其中重要的问题是,数据并不好找,因此,针对这个问题,本文也进行介绍。
本文主要针对以上三个问题的一些相关工作进行介绍,供大家一起参考。
一、泛化query多召回排序策略提升意图相关性
昨天看到一个分享,《使用RAG-Fusion和RRF让RAG在意图搜索方面更进一步》(https://mp.weixin.qq.com/s/N7HgjsqgCVf2i-xy05qZtA)介绍了《Forget RAG, the Future is RAG-Fusion》一文,(https://towardsdatascience.com/forget-rag-the-future-is-rag-fusion-1147298d8ad1),RAG-Fusion希望弥合用户明确提出的问题和他们(原本的意图)打算提出的问题之间的差距。
其思想在于通过生成多个用户查询和重新排序结果来解决RAG固有的约束;利用倒数排序融合(RRF)和自定义向量评分加权,生成全面准确的结果。 步骤很清晰,可以查看代码:
# Predefined set of documents (usually these would be from your search database)
all_documents = {
"doc1": "Climate change and economic impact.",
"doc2": "Public health concerns due to climate change.",
"doc3": "Climate change: A social perspective.",
"doc4": "Technological solutions to climate change.",
"doc5": "Policy changes needed to combat climate change.",
"doc6": "Climate change and its impact on biodiversity.",
"doc7": "Climate change: The science and models.",
"doc8": "Global warming: A subset of climate change.",
"doc9": "How climate change affects daily weather.",
"doc10": "The history of climate change activism."
}
# Main function
if __name__ == “__main__”:
original_query = “impact of climate change”
generated_queries = generate_queries_chatgpt(original_query)
all_results = {}
for query in generated_queries:
search_results = vector_search(query, all_documents)
all_results[query] = search_results
reranked_results = reciprocal_rank_fusion(all_results)
final_output = generate_output(reranked_results, generated_queries)
print(final_output)
首先,通过LLM将用户的查询转换为相似但不同的查询,例如,如果最初的查询是关于“气候变化的影响”,生成的查询可能包括“气候变化的经济后果”、“气候变化和公共卫生”等角度。
# Function to generate queries using OpenAI's ChatGPT
def generate_queries_chatgpt(original_query):
response = openai.ChatCompletion.create(
model=“gpt-3.5-turbo”,
messages=[
{“role”: “system”, “content”: “You are a helpful assistant that generates multiple search queries based on a single input query.”},
{“role”: “user”, “content”: f“Generate multiple search queries related to: {original_query}”},
{“role”: “user”, “content”: “OUTPUT (4 queries):”}
]
)
generated_queries = response.choices[0][“message”][“content”].strip().split(“n”)
return generated_queries
其次,对原始查询及其新生成的同级查询执行并发的向量搜索。
# Mock function to simulate vector search, returning random scores
def vector_search(query, all_documents):
available_docs = list(all_documents.keys())
random.shuffle(available_docs)
selected_docs = available_docs[:random.randint(2, 5)]
scores = {doc: round(random.uniform(0.7, 0.9), 2) for doc in selected_docs}
return {doc: score for doc, score in sorted(scores.items(), key=lambda x: x[1], reverse=True)}
接着,聚合和细化所有结果使用倒数排序融合(RRF),RRF是与滑铁卢大学(CAN)和谷歌(Google)合作开发,论文地址:https://plg.uwaterloo.ca/~gvcormac/cormacksigir09-rrf.pdf
具体计算公式为:
# Reciprocal Rank Fusion algorithm
def reciprocal_rank_fusion(search_results_dict, k=60):
fused_scores = {}
print("Initial individual search result ranks:")
for query, doc_scores in search_results_dict.items():
print(f"For query '{query}': {doc_scores}")
for query, doc_scores in search_results_dict.items():
for rank, (doc, score) in enumerate(sorted(doc_scores.items(), key=lambda x: x[1], reverse=True)):
if doc not in fused_scores:
fused_scores[doc] = 0
previous_score = fused_scores[doc]
fused_scores[doc] += 1 / (rank + k)
print(f"Updating score for {doc} from {previous_score} to {fused_scores[doc]} based on rank {rank} in query '{query}'")
reranked_results = {doc: score for doc, score in sorted(fused_scores.items(), key=lambda x: x[1], reverse=True)}
print(“Final reranked results:”, reranked_results)
return reranked_results
最后,将精心挑选的结果与新查询配对,引导LLM进行有针对性的查询语句输出,考虑所有查询和重新排序的结果列表。
# Dummy function to simulate generative output
def generate_output(reranked_results, queries):
return f"Final output based on {queries} and reranked documents: {list(reranked_results.keys())}"
总的来说,这种方式思路挺好的,幻觉评估问题就是采用这种思路在做,对于一个问题,找到多个回答点,作为证据,进行评估。这个文章中介绍的排序方式也有启发。但是,太慢,这是时间换效果,并且query改写成多个,多个容易漂移,而且选项太多对于排序也有影响,这个提分不明显,是优化阶段要做的事,重点要放在文本切割上。
二、基于大模型生成能力自动生成引文
在《引证生成让大模型问答结果更为可信:也看大型语言模型生成带引证的文本方法与评估基准ALCE》一文中,我们介绍了最近的工作《Enabling Large Language Models to Generate Text with Citations (使大型语言模型生成带引证的文本)》很有趣。
论文地址: https://arxiv.org/abs/2305.14627
代码地址: https://github.com/princeton-nlp/ALCE
该工作研究了一种新的LLM生成范式评测基准ALCE,给定一个问题,系统生成文本,同时从一个大型检索语料库中提供引文。每个语句可能包含多个引文(例如[1][2])
其核心工作在于如何生成引文,如VANILLA方案,向模型提供检索到的前k11段落,并指示模型进行相应的引用。
完整的指令见如表22所示:
当前,可用于引文数据集训练数据的,主流的有ASQA、QAMPARI以及ELI5,下面对其进行介绍:
1、ASQA
ASQA《ASQA: Factoid Questions Meet Long-Form Answers》是一个长格式的事实类数据集。
其中每个问题都是AmbigQA中的一个模棱两可的问题,需要多个简短答案来涵盖对问题的不同解释。
例如,问题 “美国何时脱离英国?”应同时回答1776年7月2日(独立宣言)和1783年9月3日(巴黎条约)。由于问题的模糊性,有必要综合多份文件中的信息。
地址:https://browse.arxiv.org/pdf/2204.06092.pdf
数据地址:https://github.com/google-research/language/tree/master/language/asqa
2、QAMPARI
QAMPARI《QAMPARI: A Benchmark for Open-domain Questions with Many Answers》是一个事实类QA数据集,其答案是一个实体列表,这些实体通常来自不同的段落。
该数据集利用维基百科的知识图谱和表格自动构建,问题由人工解析,答案由人工验证。该数据集代表了人们常见的 “罗列 “类型问题。
数据样例:
{
"entities":[
{
"entity_url":"https://en.wikipedia.org/wiki/Ryoichi_Ikegami",
"entity_text":"Ryoichi Ikegami",
"aliases":[
"Ryoichi Ikegami"
]
}
],
"question_text":"What manga was drawn by Ryoichi Ikegami?",
"answer_list":[
{
"answer_text":"Heat",
"aid":"799__wikidata_simple__test__0",
"aliases":[
"Heat"
],
"answer_url":"https://en.wikipedia.org/wiki/Heat_(manga)",
"proof":[
{
"proof_text":" is a seinen manga series written by buronson and illustrated by ryoichi ikegami.",
"found_in_url":"https://en.wikipedia.org/wiki/Heat_(manga)",
"pid":"799__wikidata_simple__test__0__0"
}
]
},
{
"answer_text":"Mai, the Psychic Girl",
"aid":"799__wikidata_simple__test__1",
"aliases":[
"Mai, the Psychic Girl"
],
"answer_url":"https://en.wikipedia.org/wiki/Mai,_the_Psychic_Girl",
"proof":[
{
"proof_text":"mai, the psychic girl, known simply as in japan, is a manga written by kazuya kudu014d and illustrated by ryoichi ikegami.nthe main character is mai kuju, a 14-year-old japanese girl with powerful psychic abilities.",
"found_in_url":"https://en.wikipedia.org/wiki/Mai,_the_Psychic_Girl",
"pid":"799__wikidata_simple__test__1__0"
}
]
},
{
"answer_text":"Wounded Man",
"aid":"799__wikidata_simple__test__2",
"aliases":[
"Wounded Man"
],
"answer_url":"https://en.wikipedia.org/wiki/Wounded_Man",
"proof":[
{
"proof_text":" is a japanese seinen manga written by kazuo koike and illustrated by ryoichi ikegami.",
"found_in_url":"https://en.wikipedia.org/wiki/Wounded_Man",
"pid":"799__wikidata_simple__test__2__0"
}
]
},
{
"answer_text":"Sanctuary",
"aid":"799__wikidata_simple__test__3",
"aliases":[
"Sanctuary"
],
"answer_url":"https://en.wikipedia.org/wiki/Sanctuary_(manga)",
"proof":[
{
"proof_text":" is a manga written by sho fumimura, and illustrated by ryoichi ikegami.",
"found_in_url":"https://en.wikipedia.org/wiki/Sanctuary_(manga)",
"pid":"799__wikidata_simple__test__3__0"
}
]
},
{
"answer_text":"Crying Freeman",
"aid":"799__wikidata_simple__test__4",
"aliases":[
"Crying Freeman"
],
"answer_url":"https://en.wikipedia.org/wiki/Crying_Freeman",
"proof":[
{
"proof_text":" is a japanese manga series written by kazuo koike and illustrated by ryoichi ikegami.",
"found_in_url":"https://en.wikipedia.org/wiki/Crying_Freeman",
"pid":"799__wikidata_simple__test__4__0"
}
]
},
{
"answer_text":"Strain",
"aid":"799__wikidata_simple__test__5",
"aliases":[
"Strain"
],
"answer_url":"https://en.wikipedia.org/wiki/Strain_(manga)",
"proof":[
{
"proof_text":" is a japanese manga series written by buronson and illustrated by ryoichi ikegami, published shogakukan's "big comic superior" from 1996 to 1998.nplot.nmayo is a professional assassin who is hired by the "organization" to kill the mother of a young prostitute, shion.",
"found_in_url":"https://en.wikipedia.org/wiki/Strain_(manga)",
"pid":"799__wikidata_simple__test__5__0"
}
]
}
],
"qid":"799__wikidata_simple__dev"
}
例如,”哪些电影的演员中有巩俐?为了在数据集上实现高精确度和高召回率,模型需要对多个段落进行综合,这就提出了一个独特的挑战。
地址:https://browse.arxiv.org/pdf/2205.12665.pdf
地址:https://samsam3232.github.io/qampari/
3、ELI5
ELI5《ELI5: Long Form Question Answering》是建立在Reddit论坛 “Explain Like I’m Five “6上的长式QA数据集,平均答案长度为131个单词。
大多数ELI5问题都是how/why/what问题,需要有深度的长答案和多个段落作为证据。由于问题中讨论的主题多种多样。
地址:https://aclanthology.org/P19-1346.pdf
总结
本文主要介绍了在真实落地中RAG存在几个比较突出的问题,现在也出现了从多query、多召回策略以及排序修正等多个方案,但是这些方法是以时间换效果,并且query改写成多个,多个容易漂移,而且选项太多对于排序也有影响,这个提分不明显,是优化阶段要做的事,重点要放在文本切割上。
因此,如何增强大模型自身的知识,或许才是正道?但这明显十分漫长。
参考文献
1、https://mp.weixin.qq.com/s/HFuerrV5l7h7YB7y_HzY9w
2、https://arxiv.org/abs/2305.14627
3、https://browse.arxiv.org/pdf/2204.06092.pdf
4、https://browse.arxiv.org/pdf/2205.12665.pdf
5、https://aclanthology.org/P19-1346.pdf
6、https://mp.weixin.qq.com/s/N7HgjsqgCVf2i-xy05qZtA
7、https://towardsdatascience.com/forget-rag-the-future-is-rag-fusion-1147298d8ad1
关于我们
老刘,刘焕勇,NLP开源爱好者与践行者,主页:https://liuhuanyong.github.io。
老刘说NLP,将定期发布语言资源、工程实践、技术总结等内容,欢迎关注。
对于想加入更优质的知识图谱、事件图谱、大模型AIGC实践、相关分享的,可关注公众号,在后台菜单栏中点击会员社区->会员入群加入。
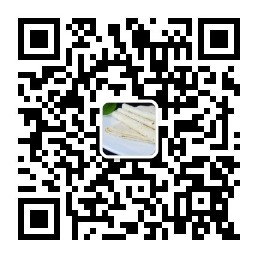