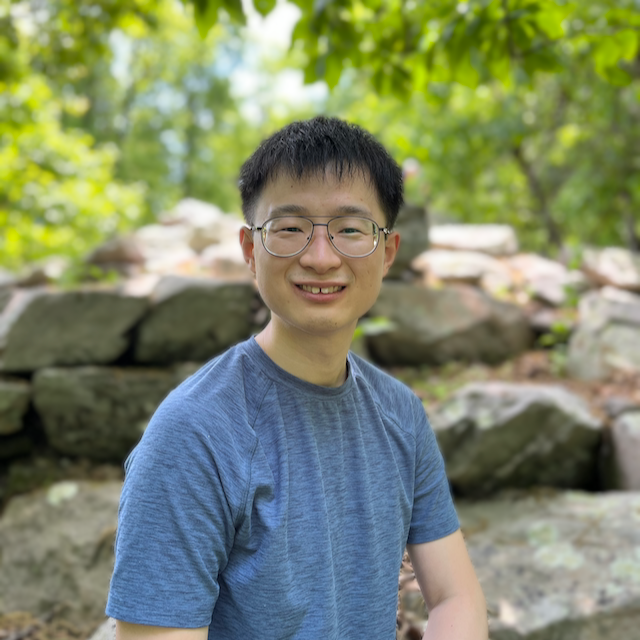
分享嘉宾吴京风
吴京风是加州大学伯克利分校西蒙斯研究所的博士后研究员,由Peter Bartlett和Bin Yu教授指导。他在约翰斯·霍普金斯大学获得计算机科学博士学位, 在北京大学获得数学硕士和学士学位。吴京风主要从事深度学习理论研究。
Jingfeng Wu is a Postdoctoral Researcher at the Simons Institute at UC Berkeley, hosted by Peter Bartlett and Bin Yu. Before that, Jingfeng completed his PhD in Computer Science at Johns Hopkins University under the supervision of Vladimir Braverman. He received his MS and BS in Mathematics from Peking University. Jingfeng is interested in deep learning theory.
分享嘉宾邹荻凡
邹荻凡博士是香港大学计算机系以及数据科学学院助理教授,博士生导师。他在美国加州大学洛杉矶分校获得博士学位,在中国科学技术大学信息与通信工程和应用物理方向获得硕士和学士学位。他的研究主要包括机器学习,深度学习理论,图神经网络,以及人工智能方法在其他领域的应用。
他在研究工作主要发表于机器学习顶会(ICML,NeurIPS,ICLR,COLT)以及国际知名期刊(IEEE trans., Nature Comm.,PNAS, etc)。他在NeurIPS,AAAI担任领域主席以及ICML,ICLR,COLT等多个会议担任审稿人。
Difan Zou is an assistant professor in computer science department and institute of data science at HKU. He has received his PhD degree in Department of Computer Science, University of California, Los Angeles (UCLA). He received a B. S degree in Applied Physics, from School of Gifted Young, USTC and a M. S degree in Electrical Engineering from USTC. His research interests are broadly in machine learning, deep learning theory, graph learning, and interdisciplinary research between AI and other subjects. His research papers have been published papers on top-tier machine learning conferences (ICML, NeurIPS, COLT, ICLR) and journals (IEEE trans., Nature Comm., PNAS, etc). He serves as the area chair/senior PC member for NeurIPS and AAAi, and PC members for ICML, ICLR, COLT, etc.
分享主题:实现线性回归中的语境学习需要多少个预训练任务?
在多样的任务上预训练的注意力模型有卓越的语境学习(in-context learning)能力,使它们能够仅基于输入上下文解决未知任务,而无需调整模型参数。我会讨论一个简化设置中语境学习的一种理论:利用线性参数化的单层线性注意力模型实现线性回归中的语境学习。我会先给出一个模型预训练的任务数量复杂性界限, 用来说明有效的预训练只需要少量的独立任务。此外,我会比较预训练的模型与贝叶斯最优算法(最佳调整的岭回归)的语境学习性能。如果在新任务中示例的数量接近预训练中示例的数量,预训练的模型与最佳调整的岭回归的语境学习性能相当。在一般情况下,预训练的模型并不能实现贝叶斯最优算法。
How Many Pretraining Tasks Are Needed for In-Context Learning of Linear Regression?
Transformers pretrained on diverse tasks exhibit remarkable in-context learning (ICL) capabilities, enabling them to solve unseen tasks solely based on input contexts without adjusting model parameters. In this talk, Jingfeng will discuss a theory of ICL in one of its simplest setups: pretraining a linearly parameterized single-layer linear attention model for linear regression with a Gaussian prior. He will first present a statistical task complexity bound for the attention model pretraining, showing that effective pretraining only requires a small number of independent tasks. Furthermore, he will compare the pretrained attention model with a Bayes optimal algorithm, i.e., optimally tuned ridge regression, in terms of their ICL performance (i.e., generalization on unseen tasks). If the number of context examples is close to that in pretraining, the ICL performance of the pretrained attention model matches that of an optimally tuned ridge regression. In general, however, the pretrained attention model does not implement a Bayes optimal algorithm.
活动时间:10月27日(周五)11:00-12:00
活动形式:线上直播,扫描下方二维码报名
关注交流:点击原文链接,还可进行线上讨论
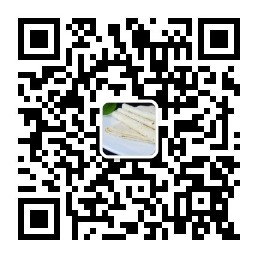