在此次演讲中,我们将分享如何赋予机器人根据物理世界做任务规划的能力。最近的很多研究表明,大型语言模型(LLMs)具有对机器人任务非常有用的知识,尤其是在推理和规划方面。然而,LLMs缺乏物理世界的grounding,同时LLMs也依赖于外部affordance模型来感知环境信息,并且这些affordance模型不能与LLMs共同进行推理。我们认为,任务规划器应该是一个统一的多模态系统。为此,我们介绍了机器人视觉-语言规划算法(ViLa),这是一种新颖的长程机器人规划方法,它利用视觉-语言模型(VLMs)生成一系列可操作的步骤。ViLa直接将感知数据整合到其推理和规划过程中,使其能够深刻理解视觉世界中的常识知识,包括空间布局和对象属性。它还支持灵活的多模态目标定义,并自然地结合视觉反馈。我们在真实机器人和模拟环境中进行的广泛评估表明,ViLa相比现在基于LLM的任务规划算法具有明显的优势,并在众多的开放世界操纵任务中取得很好的表现。
In this talk, we are interested in imbuing robots with the capability of physically-grounded task planning. Recent advancements have shown that large language models (LLMs) possess extensive knowledge useful in robotic tasks, especially in reasoning and planning. However, LLMs are constrained by their lack of world grounding and dependence on external affordance models to perceive environmental information, which cannot jointly reason with LLMs. We argue that a task planner should be an inherently grounded, unified multimodal system. To this end, we introduce Robotic Vision-Language Planning (ViLa), a novel approach for long-horizon robotic planning that leverages vision-language models (VLMs) to generate a sequence of actionable steps. ViLa directly integrates perceptual data into its reasoning and planning process, enabling a profound understanding of commonsense knowledge in the visual world, including spatial layouts and object attributes. It also supports flexible multimodal goal specification and naturally incorporates visual feedback. Our extensive evaluation, conducted in both real-robot and simulated environments, demonstrates ViLa’s superiority over existing LLM-based planners, highlighting its effectiveness in a wide array of open-world manipulation tasks.
胡英东是清华大学交叉信息研究院三年级博士生,导师为高阳教授。在此之前,他在北京邮电大学获得智能科学与技术学士学位。他的研究兴趣主要包括计算机视觉,强化学习,具身智能和机器人学习。目前专注于利用基础模型中的先验知识,构建能在开放世界泛化的通用机器人。他已经在ECCV,ICML,CoRL等多个机器学习和机器人会议上发表论文,担任ICLR,CVPR等国际学术会议审稿人。Hu Yingdong is a third-year Ph.D. student at the Institute for Interdisciplinary Information Sciences at Tsinghua University, under the supervision of Professor Gao Yang. Prior to this, he received his Bachelor’s degree in Intelligence Science and Technology from Beijing University of Posts and Telecommunications. His research interests mainly include computer vision, reinforcement learning, embodied intelligence, and robot learning. He is currently focused on using the prior knowledge in foundation models to build general-purpose robots that can generalize in the open world. He has published papers at various machine learning and robotics conferences, such as ECCV, ICML, CoRL, and serves as a reviewer for international academic conferences like ICLR and CVPR.
胡英东是清华大学交叉信息研究院三年级博士生,导师为高阳教授。在此之前,他在北京邮电大学获得智能科学与技术学士学位。他的研究兴趣主要包括计算机视觉,强化学习,具身智能和机器人学习。目前专注于利用基础模型中的先验知识,构建能在开放世界泛化的通用机器人。他已经在ECCV,ICML,CoRL等多个机器学习和机器人会议上发表论文,担任ICLR,CVPR等国际学术会议审稿人。Hu Yingdong is a third-year Ph.D. student at the Institute for Interdisciplinary Information Sciences at Tsinghua University, under the supervision of Professor Gao Yang. Prior to this, he received his Bachelor’s degree in Intelligence Science and Technology from Beijing University of Posts and Telecommunications. His research interests mainly include computer vision, reinforcement learning, embodied intelligence, and robot learning. He is currently focused on using the prior knowledge in foundation models to build general-purpose robots that can generalize in the open world. He has published papers at various machine learning and robotics conferences, such as ECCV, ICML, CoRL, and serves as a reviewer for international academic conferences like ICLR and CVPR.
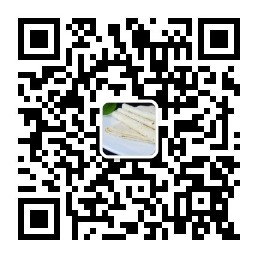