

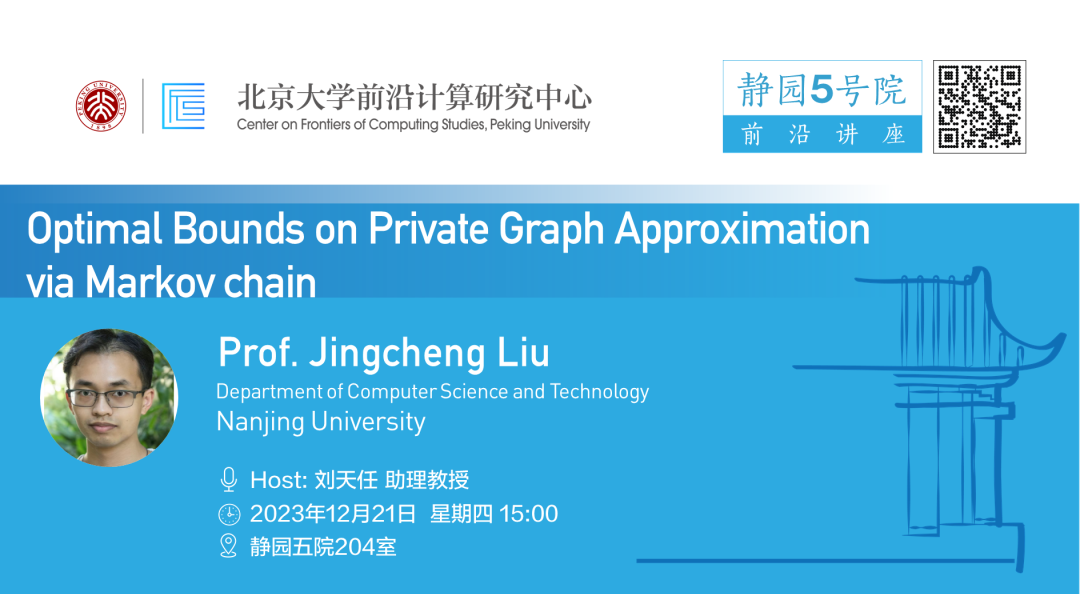
Optimal Bounds on Private Graph Approximation via Markov chain
报告人
Prof. Jingcheng Liu
Nanjing University
时间
2023年12月21日 星期四 15:00
地点
静园五院204室
Host
刘天任 助理教授
Abstract
We propose an efficient ϵ-differentially private algorithm, that given a simple weighted n-vertex, m-edge graph with a maximum unweighted degree , outputs a synthetic graph which approximates the spectrum within on the purely additive error. To the best of our knowledge, this is the first ϵ-differentially private algorithm with a non-trivial additive error for approximating the spectrum of the graph. One of the subroutines of our algorithm uses Markov chain to simulate the exponential mechanism over a non-convex set, which could be of independent interest given the recent interest in sampling from a log-concave distribution defined over a convex set. Spectral approximation also allows us to approximate all possible (S,T)-cuts, but it incurs an error that depends on the maximum degree . We further show that using our sampler, we can also output a synthetic graph that approximates the sizes of all (S,T)-cuts on n vertices weighted graph with m edges while preserving (ϵ,δ)-differential privacy and optimal additive error for weighted graphs. This removes the gap in the upper and lower bound in Eliáš, Kapralov, Kulkarni, and Lee (SODA 2020). Based on joint work with Jalaj Upadhyay and Zongrui Zou.
Biography
Jingcheng Liu is an Associate Professor in the Theory Group of the Department of Computer Science and Technology at Nanjing University. He is broadly interested in theoretical computer science, which includes randomized algorithms, computational phase transition, and differential privacy.
Before that, he completed undergrad at SJTU (ACM Honors class) and PhD at UC Berkeley, and he was a Wally Baer and Jeri Weiss postdoctoral scholar at Caltech.
往 期 讲 座
— 版权声明 —
本微信公众号所有内容,由北京大学前沿计算研究中心微信自身创作、收集的文字、图片和音视频资料,版权属北京大学前沿计算研究中心微信所有;从公开渠道收集、整理及授权转载的文字、图片和音视频资料,版权属原作者。本公众号内容原作者如不愿意在本号刊登内容,请及时通知本号,予以删除。
点“阅读原文”查看海报
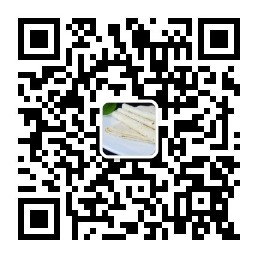