以标签为锚:从信息流动的视角分析上下文学习
Label Words are Anchors: An Information Flow Perspective for Understanding In-Context Learning
上下文学习是一种在大语言模型时代常用的小样本学习方法。它通过向大语言模型提供示例样本的方式,引导模型完成指定的任务。上下文学习无需参数更新,直观易用,非常契合大语言模型时代的需求。近来,已经有许多工作从不同角度分析了上下文学习。一些工作分析了上下文学习中示例的格式与排列组合对性能的影响,还有一些工作则尝试建立起上下文学习同梯度下降算法的联系,也有工作指出了上下文学习中任务向量的存在。我们的工作从信息流动的角度审视了上下文学习,提出并验证了“标签词在上下文学习中起锚点作用”的假设。根据这个假设,在模型的浅层,大语言模型会将示例文本中的信息汇总到相应示例的标签上,而在模型的深层,大语言模型会进一步从这些标签中提取信息来完成最终预测。本文设计了测量显著性的实验、阻断注意力的实验、测量注意力大小与分类结果相关性的实验,以验证这一猜想。进一步地,本文基于这一假设,提出了三个潜在应用:锚点重加权、仅含锚点的上下文压缩和基于锚点距离的错误诊断。这些应用展示了本文的分析结论的应用潜力,可以提高上下文学习的性能、效率,并在一定程度上解释上下文学习中出现的错误。同时,这些应用也从另一个侧面进一步证实了我们的假设。
In-context learning (ICL), a few-shot learning method pivotal in the era of large language models (LLM), guides these models using demonstration examples. This intuitive, parameter-update-free approach aligns well with the needs of the LLM era. Recent studies have explored in-context learning, focusing on the effects of demonstration format or selection, its relation to gradient descent, and the existence of task vectors in ICL. Our study examines in-context learning through the lens of information flow, introducing the ‘label words are anchors’ hypothesis. We suggest that these models initially gather information from demonstration texts onto demonstration labels in shallow layers, and then use the information aggregated on these labels for final predictions in deeper layers. We tested this hypothesis by analyzing the saliency of information flows, blocking specific flows, and linking attention magnitudes to classification outcomes. Additionally, we propose three applications based on our hypothesis: Anchor Re-Weighting, Anchor-Only Context Compression, and Anchor Distances for Error Diagnosis. These not only enhance in-context learning’s performance and efficiency but also provide insights into ICL errors and further validate our hypothesis.
王乐安,北大博士生,由孙栩老师指导。他目前的研究兴趣主要在于大模型的可解释性与机理。他在EMNLP 2023上发表的工作Label Words are Anchors: An Information Flow Perspective for Understanding In-Context Learning获得了最佳长论文奖。在此之前,他在北大图灵班(智能方向)获得了学士学位。
Lean Wang is a PhD student at Peking University, supervised by Prof. Xu Sun. His research interests lie in interpreting LLMs’ working mechanisms under various scenes. His work Label Words are Anchors: An Information Flow Perspective for Understanding In-Context Learning won the Best Long Paper Award of EMNLP 23. Previously, He received his Bachelor’s degree in Intelligence Science and Technology (Honor Track) from Turing Class, Peking University.
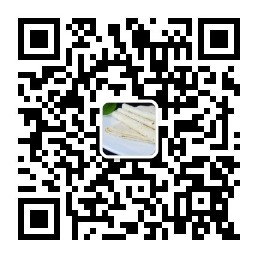